Emerging evidence underscores the profound impact of the gut microbiome, a collection of microorganisms within our digestive system, on cancer. These microorganisms collectively generate various metabolites that can significantly influence cancer progression and treatment outcomes. Dr. Zeng is employing synthetic communities and mouse cancer models to delve into the intricate connections between cancer and the microbiome. His synthetic communities, comprised of over 100 strains, allow for precise manipulation of the microbiome to elucidate the role of specific microbial metabolites in cancer. Additionally, Dr. Zeng is studying community-scale metabolism and using genetically edited strains to design synthetic communities with desired metabolic profiles. These approaches will gain valuable insights into microbiome-cancer interactions and establish a broadly applicable strategy to harness the therapeutic potential of gut microbiome. Dr. Zeng received his PhD from Princeton University, Princeton and his BS from Tsinghua University, Beijing.
All Cancers
Current Projects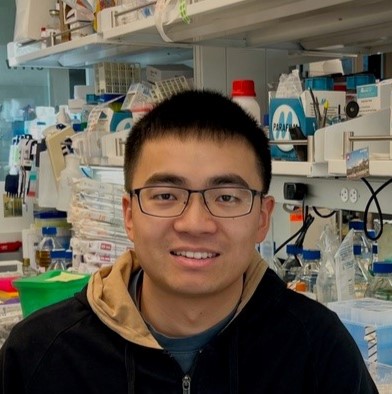

Cancer growth is often driven by the dysregulation of a class of proteins known as small GTPases. These proteins act as molecular "on/off" switches that regulate critical cellular processes such as cell division and movement. However, in cancer, these molecular switches often become stuck in the "on" state due to mutations that hamper GTP hydrolysis, the reaction that turns "off" the GTPase switch. One notable example is the family of GTPases encoded by Ras genes, which are mutated in 30% of all human cancers. Dr. Zhang's research aims to design small molecules that inactivate these mutant GTPases by accelerating GTP hydrolysis. His research will provide a new therapeutic mechanism for the treatment of mutant Ras-driven cancer for which no direct therapies are yet available. The design principles may also apply to the modulation of other small GTPases whose overactivation underlies cancer progression.
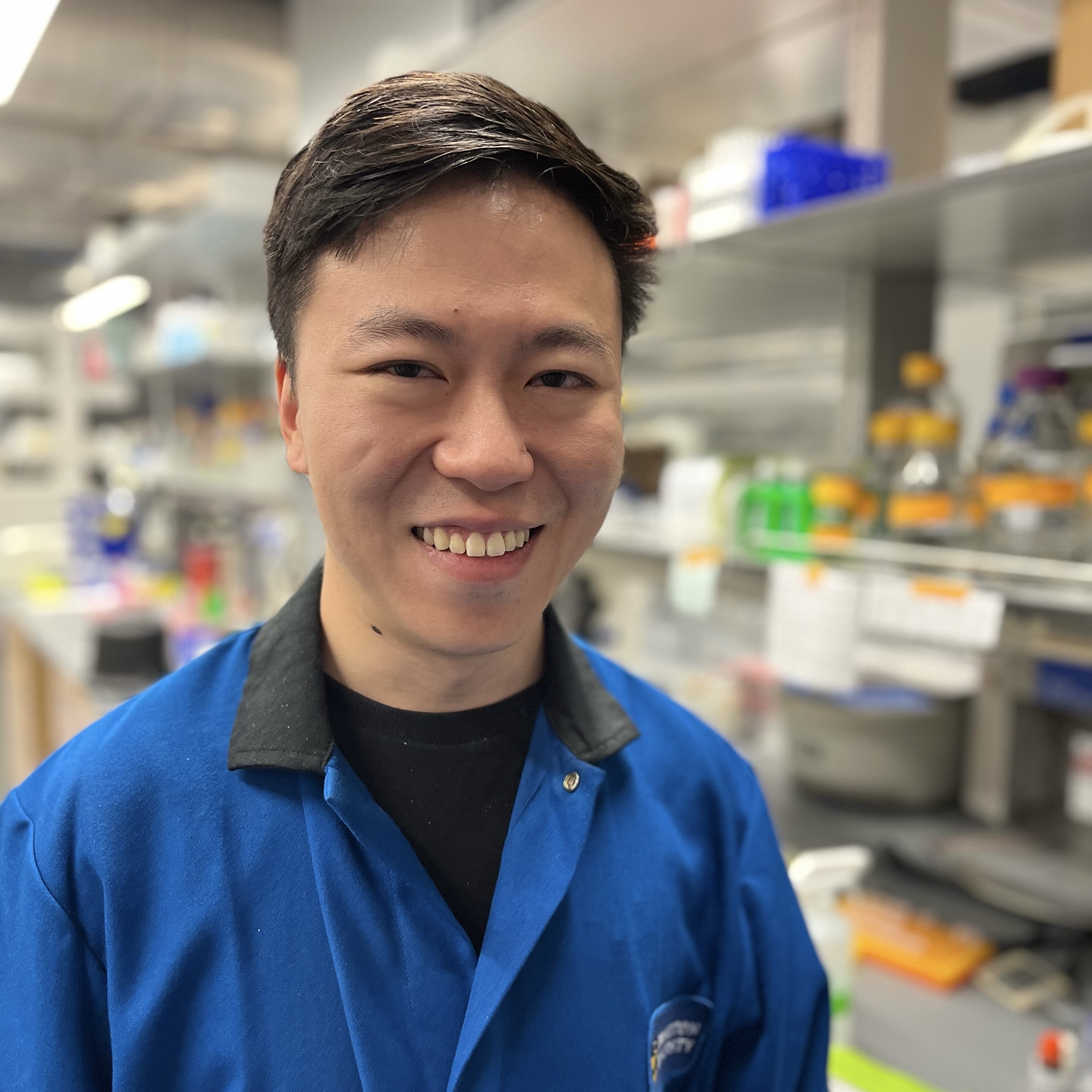
In cells, DNA wraps around a protein complex consisting of proteins called histones. Chemical modifications to histones can affect gene expression, which is key to activating or suppressing cancer progression. Histone monoaminylation, in which an amine (e.g., serotonin, dopamine, or histamine) attaches itself to a histone, is a newfound type of epigenetic modification whose role remains elusive in these processes. Dr. Zhang is using chemical biology tools to study the functions of these modifications as well as their effects on other adjacent, pre-existing cancer-associated modifications. This research may establish a foundation for how this epigenetic modification regulates gene expression and offer insight into the role of amines in the progression of cancer and human neurodegenerative disorders. Dr. Zhang received his PhD from the California Institute of Technology, Pasadena and his BS from Tsinghua University, Beijing.
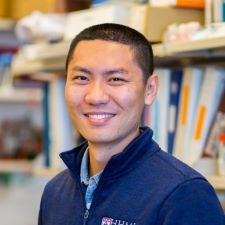
On the cellular level, aging manifests as cellular senescence—when cells permanently stop multiplying but do not die. Aberrant accumulation of senescent cells is thought to be a major contributor to age-dependent tissue degeneration and its associated pathologies. Elimination of senescent cells has been shown to improve age-associated tissue damage pathologies and extend healthy lifespan in mice. Senescent cells undergo extensive remodeling on their surface, including increased production of many surface proteins. Dr. Zhang is using a quantitative proteomics approach to investigate the mechanisms and biological consequences of cell surface remodeling in senescent cells. His goal is to identify new therapeutic targets on the senescent cell surface and develop next-generation chimeric antigen receptor (CAR) T cells and antibodies to evaluate their impact on age-related diseases. Success with this approach may have a transformative impact on treating life-threatening diseases like cancer, fibrosis, and atherosclerosis. Dr. Zhang received his PhD from Gerstner Sloan Kettering Graduate School and his BS from Sun Yat-Sen University.
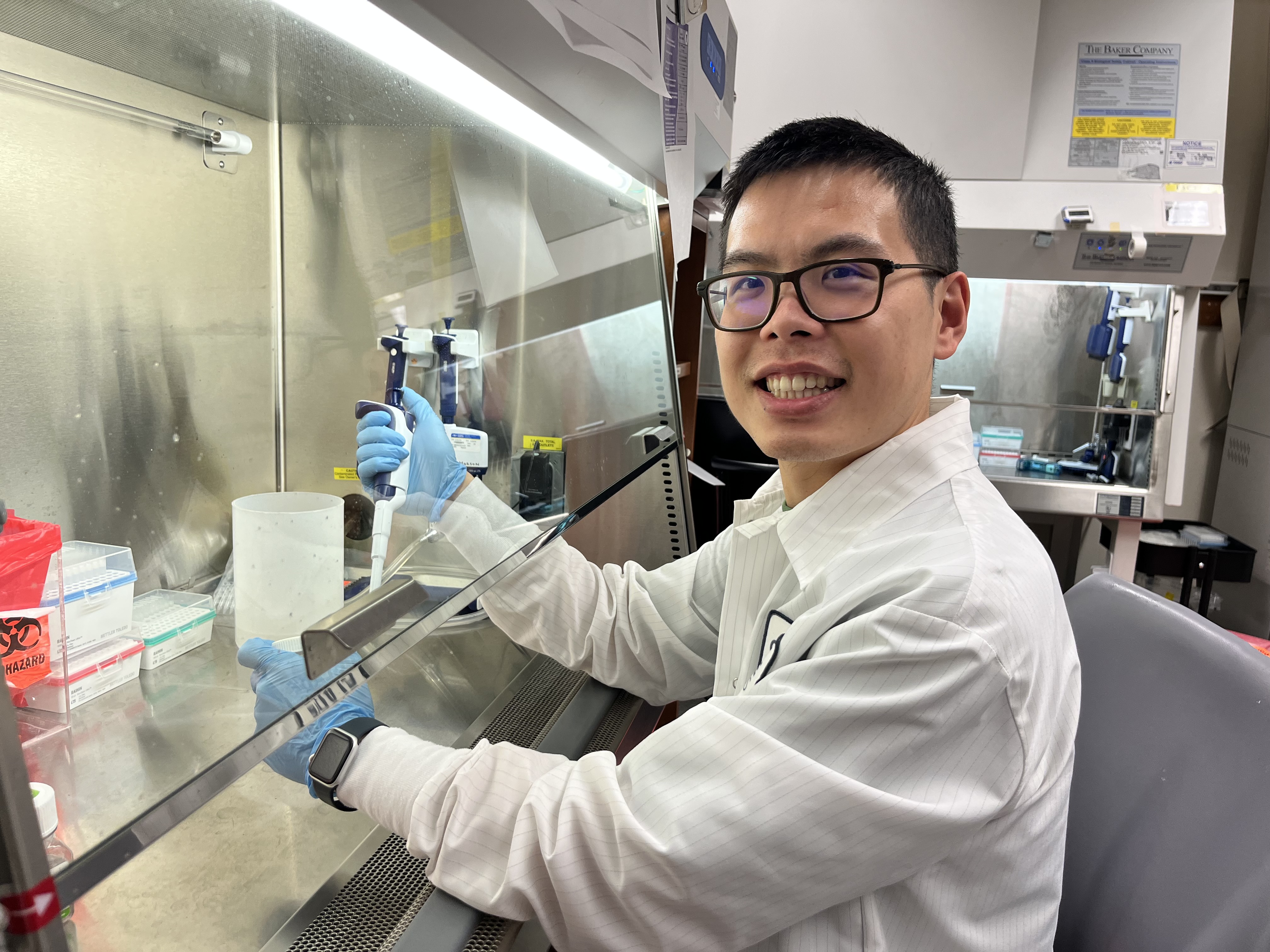
Our immune system can help us prevent or slow cancer development. Human CD4+ T cells play critical roles in regulating our immune responses to fight cancer. Upon encountering a pathogen, naïve CD4+ T cells differentiate into different T helper (Th) cells to perform diverse immune-modulatory functions. Variability in this differentiation process is associated with variable responses to cancer immunotherapy. While several genes necessary for differentiation have been identified, researchers lack a comprehensive map and a predictive model of the larger gene regulatory network (GRN) controlling this process. Dr. Zhu [Connie and Bob Lurie Fellow] plans to combine functional genomics with mathematical modeling to systematically map and model the human CD4+ T cell differentiation GRN and use the GRN model to predict and control the differentiation process. His work promises to provide a quantitative understanding of the CD4+ T cell differentiation process and open up new strategies for safer and more effective cell-based cancer therapy. Dr. Zhu received his PhD from the California Institute of Technology, Pasadena and his BS from Hong Kong University of Science and Technology, Hong Kong.
Dr. Zweig is modeling how gene regulation changes across time and space using advanced geometry, deep learning models, and CRISPR gene-editing technology. With these tools, it is possible to learn how RNA expression evolves in a cell over time and, ideally, predict evolution in regions susceptible to cancerous mutation(s). This work has the potential to apply to many types of cancer but is especially applicable for acute myeloid leukemia (AML). This is in part because there are known “precursor” states—when cells are at higher risk of becoming cancerous—where understanding gene regulation is most impactful, and in part because a common element of AML treatment is a stem cell transplant, which may attack host cells inconsistently in different regions of the same organ.
The project models temporal gene dynamics via stochastic differential equations, parameterizing the drift vector field with linear networks or shallow neural networks to guarantee provable identifiability, trained via differentiation through the adjoint method. Spatial interactions between spot clusters are characterized with graph neural networks, symmetric functions parameterizing summary statistics, and self-attention modules on latent gene embeddings. The latent embeddings are also defined through a variational autoencoder integrating RNA with other modalities.